class: center, middle # Boosting CS534 - Machine Learning Yubin Park, PhD --- class: center, middle Recall our original problem: $$ \min_{f} \mathcal{L}(\mathbf{y}, f(\mathbf{X}))$$ As the original problem is difficult to solve, we limited our search as follows: $$ \min_{\mathbf{\beta}} \mathcal{L}(\mathbf{y}, f(\mathbf{X};\mathbf{\beta}))$$ (Remember the linear models) --- class: center, middle This time, let's try to solve the difficult one directly. Perhaps, we can try [Gradient Descent](https://en.wikipedia.org/wiki/Gradient_descent) on the function itself? $$ \mathbf{f}\_k = \mathbf{f}\_{k-1} - \rho\_k \frac{\partial \mathcal{L}(\mathbf{y}, \mathbf{f})}{\partial \mathbf{f}} \Bigr|\_{\mathbf{f}=\mathbf{f}_{k-1}} $$ where `\(\mathbf{f} = [f(\mathbf{x}_1), f(\mathbf{x}_2), \cdots, f(\mathbf{x}_n)]^T\)` --- class: center, middle Note that we are looking at $$ \frac{\partial \mathcal{L}(\mathbf{y}, \mathbf{f})}{\partial \mathbf{f}} $$ not $$ \frac{\partial \mathcal{L}(\mathbf{y}, f(\mathbf{X}; \mathbf{\beta}))}{\partial \mathbf{\beta}} $$ --- ## Meaning of the Functional Gradient Consider Squared Loss for example. $$ \frac{\partial \mathcal{L}(\mathbf{y}, \mathbf{f})}{\partial \mathbf{f}} = \frac{1}{2}\frac{\partial (\mathbf{y}- \mathbf{f})^T(\mathbf{y}- \mathbf{f})}{\partial \mathbf{f}} = -(\mathbf{y} - \mathbf{f}) $$ Plugging the above into the update form: $$ \mathbf{f}\_k = \mathbf{f}\_{k-1} + \rho\_k (\mathbf{y} - \mathbf{f}_{k-1})$$ If we initialize `\(\mathbf{f}_0 = \mathbf{0}\)`, then $$ \mathbf{f}\_1 = \mathbf{f}\_{0} + \rho\_1 (\mathbf{y} - \mathbf{f}_{0}) = \rho\_1 \mathbf{y} $$ $$ \mathbf{f}\_2 = \mathbf{f}\_{1} + \rho\_2 (\mathbf{y} - \mathbf{f}_{1}) = (\rho\_1 + \rho\_2 - \rho_1\rho\_2 )\mathbf{y}$$ $$ \vdots $$ $$ \lim_{k \rightarrow \infty} \mathbf{f}_k = \mathbf{y}$$ --- ## Approximating the Functional Gradient (1) This seems too obvious and not that useful. In the end, we need a function that can process **unseen** data. One idea is to **approximate** the gradient with another function: $$ \frac{\partial \mathcal{L}(\mathbf{y}, \mathbf{f})}{\partial \mathbf{f}} \Bigr|\_{\mathbf{f}=\mathbf{f}\_{k-1}} \approx h\_{k} (\mathbf{X})$$ where `\(h_{k} (\mathbf{X})\)` is a function that maps `\(\mathbb{R}^{n \times m}\)` to `\(\mathbb{R}^{n }\)`. For example, if `\(h(\mathbf{X}) = \mathbf{X}\mathbf{\beta}\)` (a linear regression), $$ \mathbf{y} - \mathbf{f}\_{k-1} \approx \mathbf{X}\mathbf{\beta}_{k}$$ $$ \mathbf{f}\_k = \mathbf{f}\_{k-1} + \rho\_k \mathbf{X}\hat{\mathbf{\beta}}_{k} $$ Similar to **Forward Stagewise Regression**? --- ## Approximating the Functional Gradient (2) After `\(K\)` iterations, you have $$ \mathbf{f}\_K = \mathbf{f}\_0 + \sum_{k=1}^K \rho_k h_k(\mathbf{X})$$ For a new dataset, `\( \mathbf{X}^{\prime} \)`, you can use this collection of functions to predict the target variable: $$ \hat{\mathbf{y}} = \mathbf{f}\_0 + \sum_{k=1}^K \rho_k h_k(\mathbf{X}^{\prime}) $$ --- ## Gradient Boosting Machine 1. Initialize `\( \mathbf{f}_0 = \arg \min_{\gamma} \mathcal{L}(\mathbf{y}, \gamma) \)` 2. For `\(k=1\)` to `\(K\)`: 1. Set `\( \mathbf{r}_k = - \frac{\partial \mathcal{L}(\mathbf{y}, \mathbf{f})}{\partial \mathbf{f}} \Bigr|_{\mathbf{f}=\mathbf{f}_{k-1}} \)` 2. Fit `\(h_k(\mathbf{X})\)` to `\(\mathbf{r}_k\)` 3. Find `\(\rho_k\)` that minimizes the loss function 4. Update `\(\mathbf{f}_k = \mathbf{f}_{k-1} + \rho_k h_k(\mathbf{X})\)` `\(h(\mathbf{X})\)` can be any parametric or non-parametric function. Decision Tree is a popular choice for `\(h(\mathbf{X})\)`. When Decision Tree is used as `\(h(\mathbf{X})\)`, then you can estimate `\(\rho_k\)` for each region (or node) of the estimated decision tree. This variant of Gradient Boosting Machine (GBM) is called **[TreeBoost](https://projecteuclid.org/euclid.aos/1013203451)**. --- ## GBM for Classification $$ \mathcal{L}(\mathbf{y}, f(\mathbf{X})) = - \sum_{i=1}^{n} [ y_i f(\mathbf{x}_i) - \log (1 + \exp(f(\mathbf{x}_i)))] $$ Note that, for Logistic Regression, we have `\( f(\mathbf{x}_i) = \mathbf{x}_i\mathbf{\beta} \)`. Taking the first derivative w.r.t. the function: $$ - \frac{\partial \mathcal{L}(\mathbf{y}, \mathbf{f})}{\partial \mathbf{f}} = [y_1 - p_1, y_2 - p_2, \cdots, y_n - p_n ]^T$$ where `\( p_i = \frac{1}{1 + \exp(-f(\mathbf{x}_i))} \)`. --- ## Stochastic Gradient TreeBoost GBM can fit the training data very well, sometimes too well. To mitigate overfitting to the training data, [Stochastic Gradient TreeBoost (SGTB)](https://statweb.stanford.edu/~jhf/ftp/stobst.pdf) adds two regularization mechanisms: - **Shrinkage**: when updating the function, we reduce the contribution of each model by a factor `\(0< \nu < 1\)`. Thus, the update becomes: $$ \mathbf{f}\_k = \mathbf{f}\_{k-1} + \nu \rho_k h_k(\mathbf{X}) $$ - **Subsampling**: to reduce the variance (in the bias-variance trade-off), at each iteration, we fit a model on randomly subsampled data points. Typically 50% to 70%. --- class: middle, center 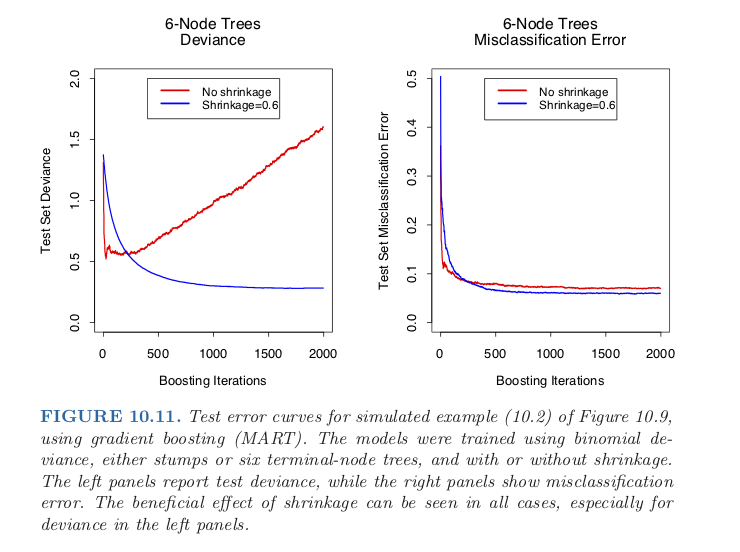 .reference[Chapter 10 of [ESLII](https://web.stanford.edu/~hastie/ElemStatLearn/)] --- class: middle, center ** IMPORTANT ** Please read the Chapter 10.14 of [ESLII](https://web.stanford.edu/~hastie/ElemStatLearn/). --- class: center, middle ## Questions?